Guide: How to Add Lora With Weight Stable Diffusion?
In the ever-evolving landscape of machine learning, incorporating cutting-edge techniques is paramount. One such innovation that has gained significant attention is the fusion of multiple Lora with Weight Stable Diffusion.
In this guide, we’ll explore the intricacies of this integration, offering a step-by-step approach to enhance your model’s performance.
Also read: Does Circle K Accept EBT?
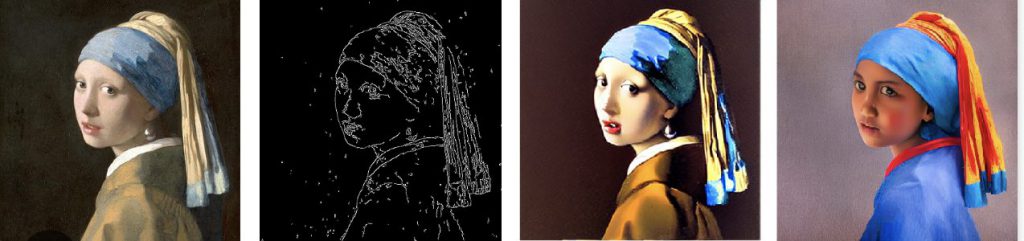
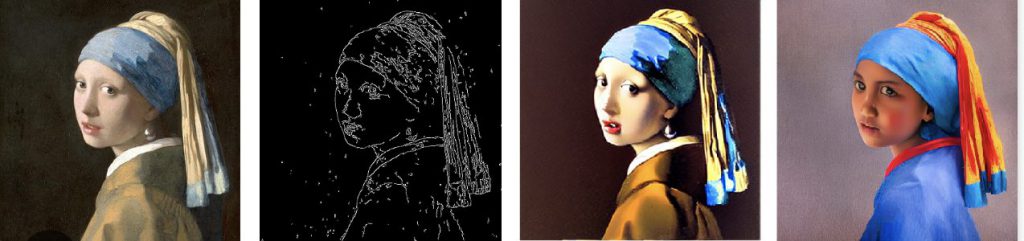
Understanding the Essence: Lora and Stable Diffusion
Decoding Multiple Lora
With its prominence in various machine learning applications, Lora brings a unique set of capabilities.
Integrating multiple Lora allows for a more nuanced approach to understanding complex patterns and generating diverse outputs.
As we embark on this journey, it’s crucial to comprehend the synergy between Lora and the Stable Diffusion model.
Unpacking Weight Stable Diffusion
Stable Diffusion, a model known for its stability in generating high-quality images, forms the cornerstone of our exploration.
By introducing weights to the Stable Diffusion model, we aim to fine-tune its performance, achieving a delicate balance that enhances both precision and versatility.
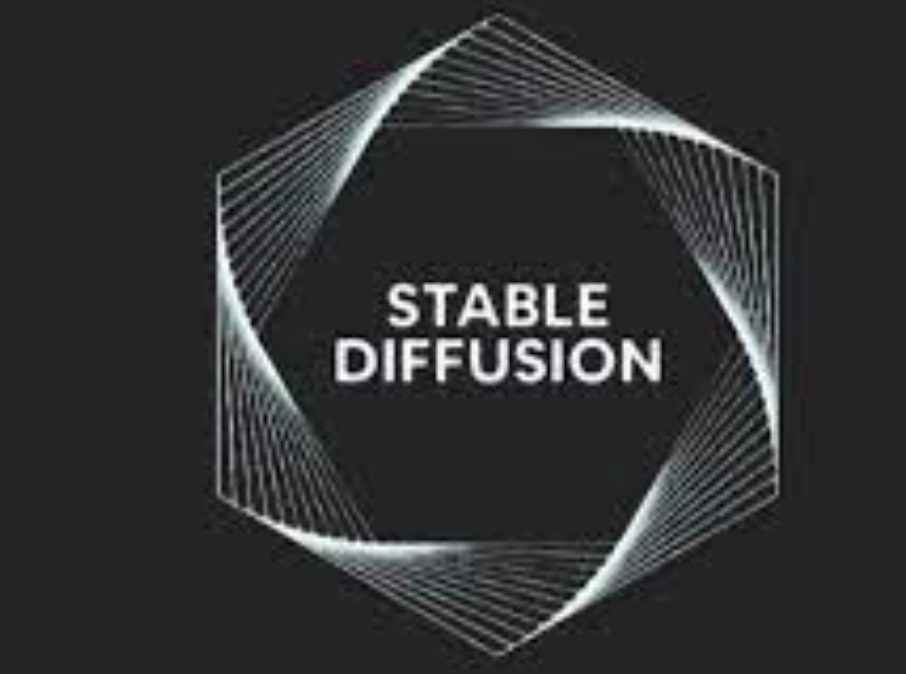
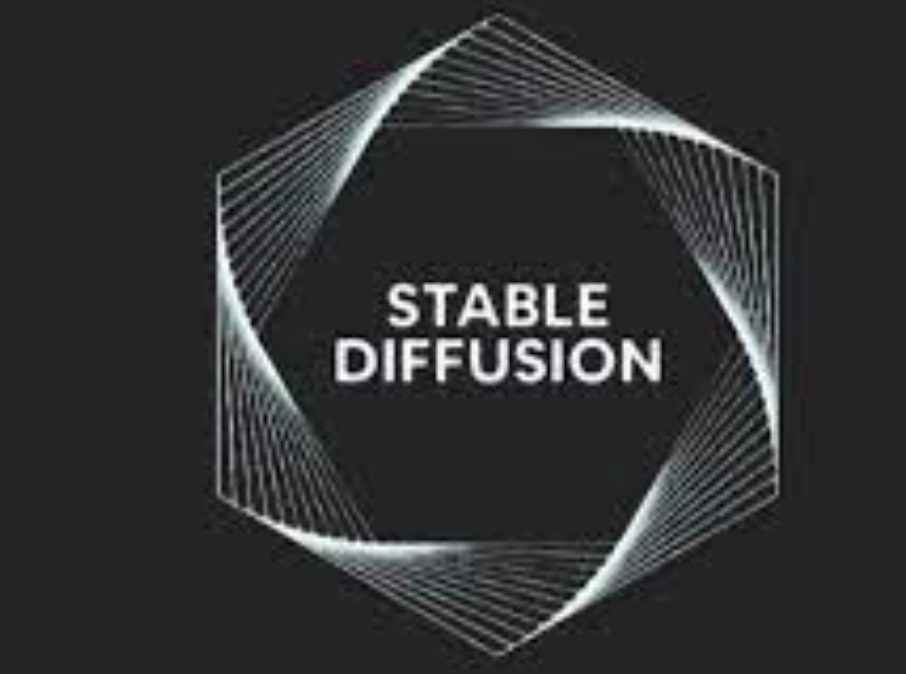
Navigating the Integration
Initiating the Fusion: Fine-Tuning Stable Diffusion Models with Lora
The integration process begins with the fine-tuning of Stable Diffusion models using multiple Lora. This involves adjusting the weights, a critical step in achieving a harmonious collaboration between the models.
As you delve into the codebase, ensure that you’re working with Stable Diffusion v1.5 or a later version to harness the advancements in the model.
The Role of Batch Size and Cross-Attention Layers
Optimizing the batch size becomes pivotal in handling the amalgamation of Lora with Stable Diffusion. Adjusting this parameter contributes to the model’s efficiency and performance.
Additionally, incorporating cross-attention layers enhances the model’s ability to focus on relevant features, fostering more accurate and context-aware image generation.
Fine-Tuning for Precision
Checkpoint Models for Seamless Progress
Regularly creating checkpoints for your models throughout the integration process ensures that you have a fallback in case of unexpected errors or changes.
This step is crucial, especially when dealing with experimental enhancements like integrating multiple Lora with Weight Stable Diffusion.
Navigating Text Error and Text Encoder Challenges
Fine-tuning Stable Diffusion models with Lora may encounter challenges related to text errors and text encoders.
Pay careful attention to these aspects, adjusting parameters and configurations to address any discrepancies and refine the model’s understanding of textual input.
Leveraging Lora Weights: A Closer Look
Harnessing the Power of Lora Weights
Integrating Lora with Stable Diffusion necessitates a detailed consideration of Lora weights. These weights are pivotal in influencing the model’s decisions and outputs.
Experiment with different weight configurations to strike a balance that aligns with your specific use case and objectives.
Also read: BRICS: Russia & India Partner to Create a Digital Economy
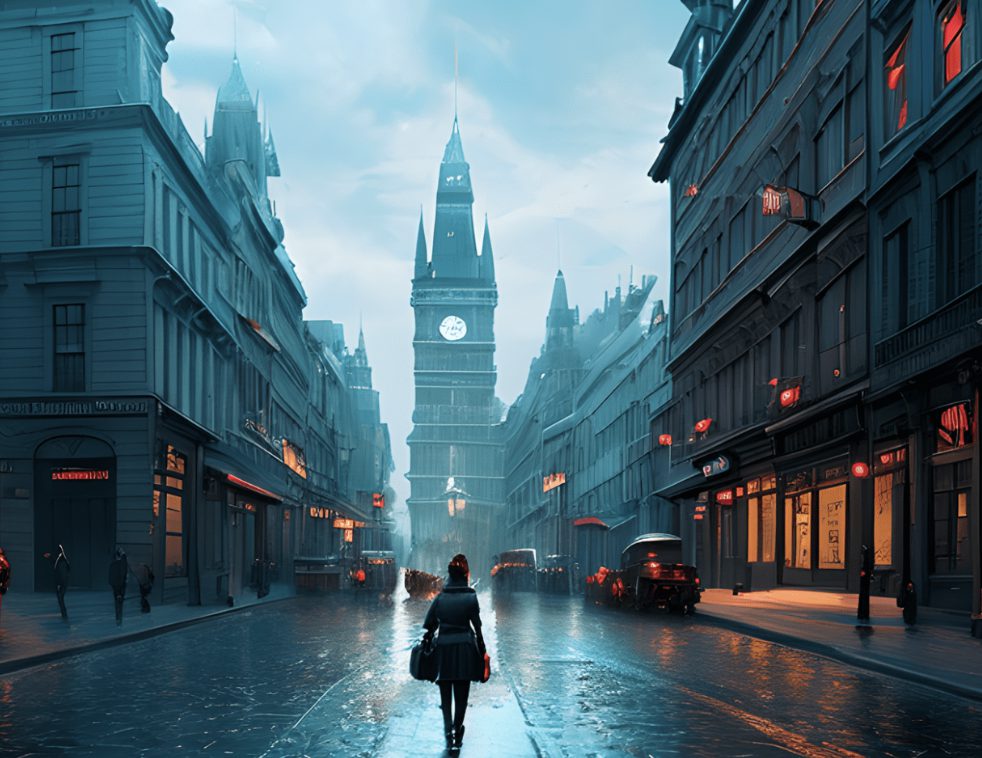
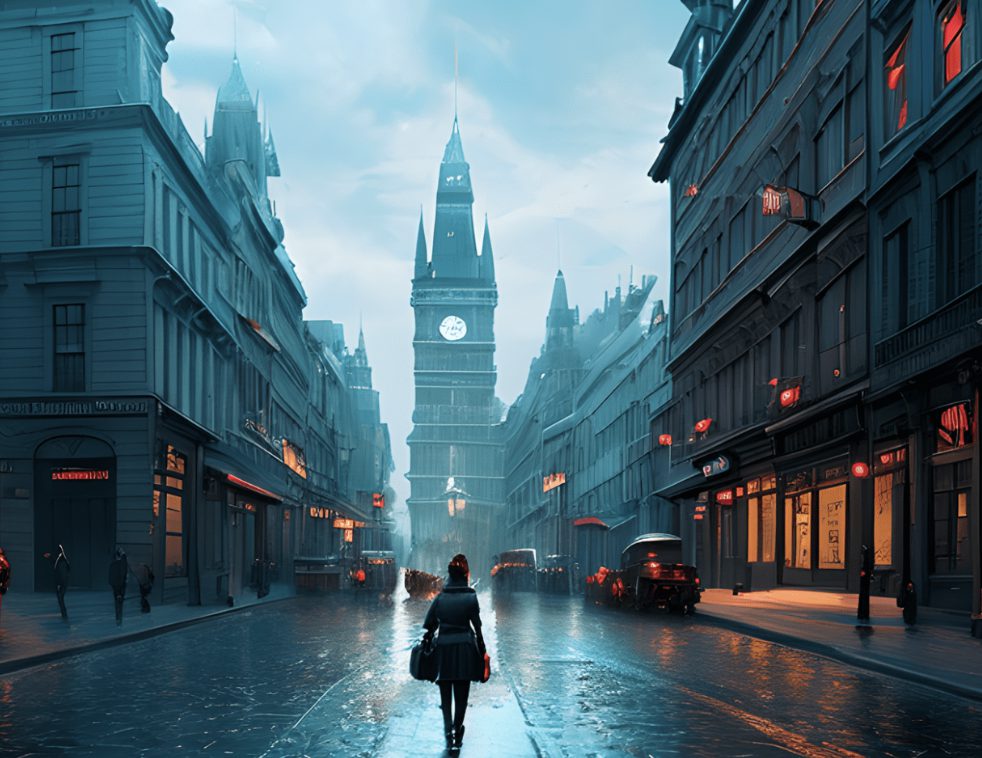
The Original Model vs. The Enhanced Model
As you progress with the integration, periodically compare the outputs of the original Stable Diffusion model with the enhanced version, incorporating multiple Lora.
This comparative analysis provides valuable insights into the impact of the integration and guides further adjustments for optimal performance.
Embracing the Future: A Model Fine-Tuned
Unveiling the Potential of Lora Trained Stable Diffusion
Upon successful integration, the model emerges as a powerful tool with enhanced capabilities in image generation and pattern recognition.
The marriage of Lora with Weight Stable Diffusion elevates the model’s proficiency, making it adept at handling diverse tasks in the realm of machine learning.
Implications and Applications
The implications of integrating multiple Lora with Weight Stable Diffusion are vast. The fine-tuned model opens avenues for innovative applications across various domains, from creative image generation to sophisticated pattern recognition.
As you explore these possibilities, consider your advanced model’s ethical implications and responsible usage.
In Conclusion: Pioneering the Next Wave
In conclusion, integrating multiple Lora with Weight Stable Diffusion represents a significant stride in the evolution of machine learning models.
By navigating the intricacies of fine-tuning and leveraging the collaborative power of Lora, you embark on a journey that pioneers the next wave of innovation in the field.