How to Train Stable Diffusion?: A Comprehensive Guide
Welcome to Stable Diffusion, where machine learning and artificial intelligence combine to produce stunning images. In this comprehensive guide, we’ll embark on a journey to demystify the intricacies of training Stable Diffusion models.
From Google Colab prowess to the nuances of model design, let’s explore the steps to hone your skills in generating captivating images.
Unveiling the Essentials of Stable Diffusion Training
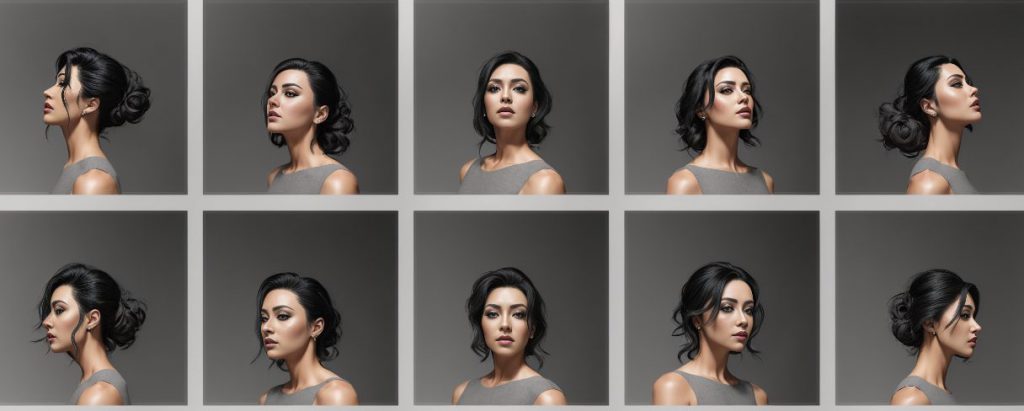
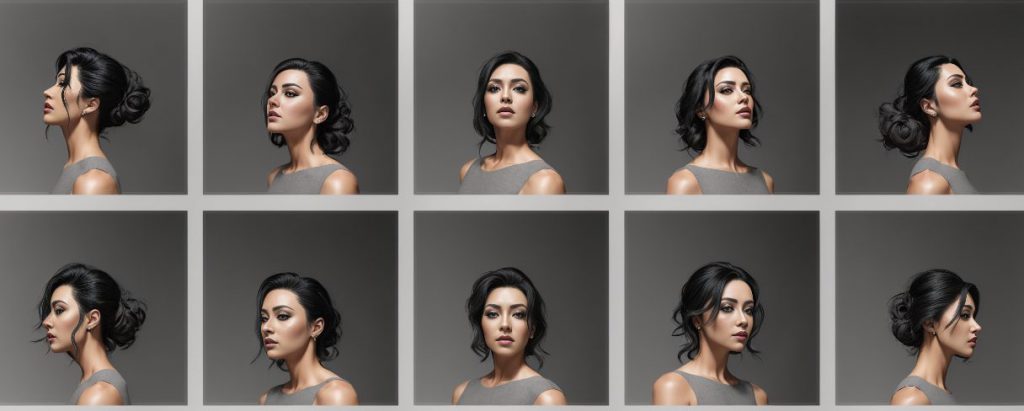
Understanding the Basics
Before delving into the training process, it’s essential to grasp the fundamentals. Stable diffusion operates on generating images by gradually refining random noise.
This process, guided by neural networks, results in the creation of intricate and high-quality final images.
The Role of Google Colab
Google Colab emerges as a powerful ally in your Stable Diffusion journey. Leveraging the capabilities of this cloud-based platform allows you to harness the prowess of neural networks without the need for hefty computing resources.
The collaborative nature of Google Colab ensures seamless exploration and execution of your training endeavors.
Also read: BRICS: India Gets Reality Check, Ditching US Dollar Becomes Impossible.
Initiating the Training Process
Preparation of Training Images
The foundation of a successful Stable Diffusion model lies in the quality and diversity of training images. Curate a dataset that encapsulates the visual richness you aim to achieve.
This dataset should be extensive, covering a spectrum of scenarios to impart versatility to your model.
Model Input: From Random Noise to Masterpieces
As you train, the model ingests random noise as its starting point. This seemingly chaotic input becomes the raw material from which your Stable Diffusion model will craft its visual symphony.
The infusion of random noise initiates the journey toward generating images that mirror the essence of your training dataset.
Model Evaluation: Navigating the Path to Excellence
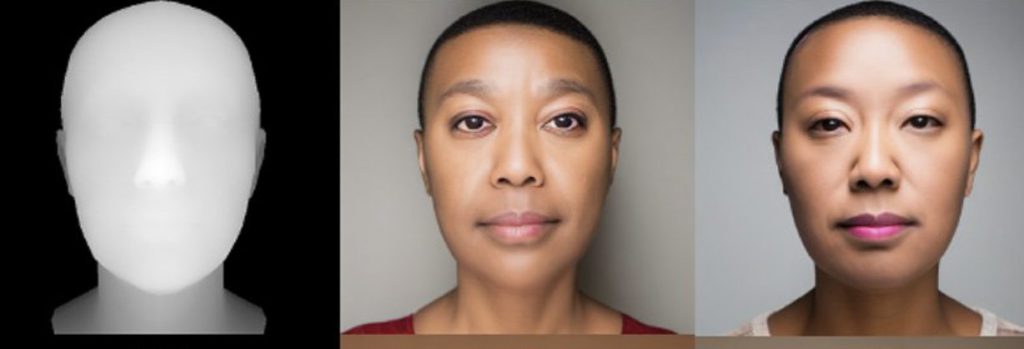
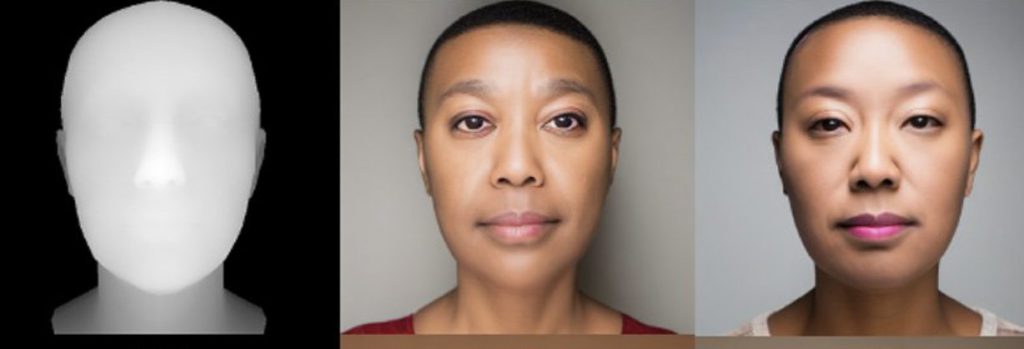
Fine-Tuning and Evaluation
Training a Stable Diffusion model is an iterative process. Periodically evaluate the model’s progress to fine-tune its parameters. This evaluation involves assessing the generated images against your dataset, ensuring the model converges toward your desired visual output.
Large Datasets and Model Robustness
The power of large datasets manifests in the robustness of your model.
Exposure to diverse scenarios equips the model to handle different input patterns, creating final images that exhibit a heightened level of detail and realism.
Crafting a Stable Diffusion Model: Design Considerations
Strategic Model Design
The architecture of your Stable Diffusion model is a pivotal factor in its performance. Strategic design considerations involve configuring the number of layers, the dimensionality of input images, and the intricate details that define the neural network’s structure.
Balancing complexity with efficiency is the key to crafting a model that generates exceptional-quality images.
Optimizing Training for Efficiency
Efficiency is paramount in the training process. Leveraging techniques such as layer normalization and strategic initialization of weights contributes to a smoother convergence of your Stable Diffusion model.
These optimizations ensure that your model navigates the training landscape precisely and quickly.
Final Steps: Bringing Your Model to Life
Generating Final Images
As your model evolves through the training epochs, the magic unfolds in generating final images. These images encapsulate the essence of your dataset, transformed into a visual tapestry by the neural networks at play.
The culmination of your training efforts manifests in images that mirror the diversity and richness of your initial dataset.
Continuous Learning and Refinement
The journey doesn’t end with the generation of final images. Embrace a culture of continuous learning and refinement.
Iteratively evaluate your model’s performance, introduce new training images, and explore advanced model architectures to push the boundaries of what your Stable Diffusion model can achieve.
Conclusion: Elevating Your Stable Diffusion Expertise
In conclusion, training Stable Diffusion models is an art form that harmonizes machine learning principles and the creative realm of image generation.
From Google Colab proficiency to the strategic design of your model, each step contributes to the evolution of a powerful and versatile image-generating entity.
As you venture into the world of Stable Diffusion, let this guide be your compass, guiding you toward mastery and innovation in artificial intelligence and visual creativity.